Secure Data Is FAIR(er) Data
Three Steps to Realize the Full Potential of Data as Assets in Biopharma Research and Development
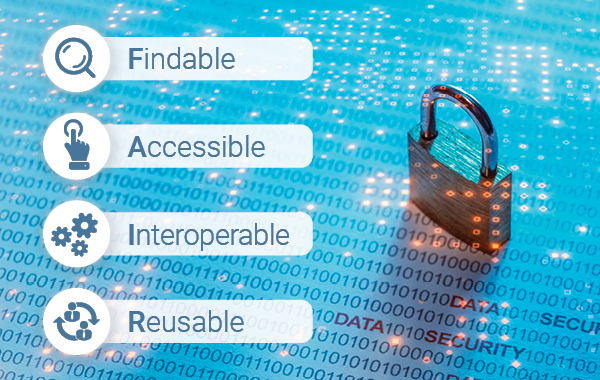
Author: Sebastian Ribi Ph.D., Scientific Business Consulting, Genedata
R&D data is a strategic asset for biopharmaceutical companies, which is utilized to build a more accurate and meaningful picture of targets, molecules, patients and their responses to drugs.
The FAIR data principles, which we explain in Step 2, below, have been adopted by many companies across all industries, including biopharma organizations around the globe.
However, in biopharma, making data FAIR is not sufficient. Based on our experience in deploying Genedata Profiler as institutional memory for all translational and clinical data, our clients require an IT and software solution which complies with strict regulatory and security regulations for working with sensitive patient data.
Step 1: Break Down Data Silos
While highly specialized drug development units are of tremendous value in an industry that relies heavily on expertise across the value chain, data generated by each function within a given company often remains siloed. These data silos are a big challenge for companies that need to federate their distributed data effectively to increase clinical trial success rates.
Biopharma companies are increasingly recognizing the value of moving away from owning and using data once for a limited purpose, toward sharing and reusing data for any number of analyses. This interdisciplinary integration of data can only be achieved by breaking down these data silos. And the biopharma industry is a perfect candidate for such a translational approach of using and sharing data.
Every step in the drug development process, including each phase in drug discovery, preclinical research, and clinical development, provides useful gates for important evaluations. Using the right data and enabling efficient and effective collaboration between a company’s talents helps identify the ‘5Rs’ for any given indication—i.e. right target, right tissue, right safety, right patient, and right commercial potential. This can improve the success rate from drug nomination to Phase III completion tremendously.
Ultimately, breaking down data silos helps companies build an institutional memory for all their data and make data-informed decisions. For biopharma, this means fostering early go/no-go decisions, which will slash drug development times and vastly reduce the number of costly late-stage failures.
Step 2: Make Data FAIR
Biopharmaceutical R&D is a complex and interdisciplinary process. Typically, R&D data originate from a variety of sources, including internal databases, external collaborations, and public repositories. Once the infrastructure is in place to bring all this data together, it is crucial to implement the FAIR guiding principles for scientific data management and stewardship in order to fully utilize the power of this data. Making data FAIR (i.e. Findable, Accessible, Interoperable, and Reusable) is what further amplifies the value of data assets.
Data users who can easily find and access the relevant data they need to answer a specific scientific question can generate new insights quickly. And these insights are even more valuable when they derive from integrated data sets that interoperate with applications for storage, processing, and analysis. But the ultimate goal of data FAIRification is to maximize data reuse. To achieve this, new data management approaches are required to centralize and massively harmonize the enormous amount of R&D data. This is what provides human access to high-quality integrated data and digital technologies.
Data FAIRification is not an easy task. A key challenge is to establish the right infrastructure that takes into account the data volume and privacy of patient-level information. Biopharmaceutical companies are increasingly recognizing the value of software providers that specialize in solutions for R&D data. An essential aspect of these platform offerings must be to increase data FAIRness by having one consistent set of requirements that are applied to all data sets. This enables scaling with the many ongoing digitalization projects in biopharmaceutical R&D. Only if data FAIRification advances can the power of artificial intelligence (AI), which relies on massive amounts of harmonized, good quality data, truly be exploited. And moving to the AI space holds so much promise for the development of next-generation therapeutics.
Biopharma organizations therefore need to move rapidly from focusing on fragmented assets to become holistic, collaborative enterprises that make data-informed decisions by breaking down data silos and making data FAIR.
Step 3: Keep Data Secure
Biopharmaceutical R&D generates large amounts of patient-related information, ranging from low-dimensional clinical to high-dimensional multi-omics data. Hence, just making data FAIR is not enough. Digital platforms that scale with the massive volume of data generated in clinical trials nowadays are needed.
And above all, these platforms must keep sensitive, patient-level data secure. This is particularly important for clinical trials that must comply with informed consent policies. Subjects who take part in clinical investigations can withdraw their consent at any time. This must be addressed by the platforms used to store, process, and analyze individual patient-level data—ideally automated to reduce human error and ensure data privacy. In addition, researchers must only have access to data to which they are entitled and a platform that serves as the single source of truth for all data must deliver this level of security.
Therefore, data access should be controlled according to project memberships and system user roles, and ideally finely graded with permissions for specific data types and sets. Data governance must be fulfilled by ensuring high quality and traceability throughout the data life cycle, a prerequisite for biopharma organizations that perform research in regulatory-regulated environments.
For the biopharma industry this means implementing a data infrastructure that not only maintains FAIR data, but also keeps patient-level data secure. Genedata Profiler offers an enterprise software solution that breaks down data silos and works as the single source of truth for all translational and clinical research data, keeping data secure and FAIR.
Download as a PDF