Machine Learning and Data Mining Techniques for Phenotypic Screening
SLAS High-Content Screening Conference, Dresden, Germany
June 29, 2016
Analysis of image-based high content screens typically starts with automated image analysis followed by processing and analysis of the extracted numerical data. This data analysis workflow often consists of three canonical steps: a) ensuring result integrity through appropriate QC procedures and result comparability through data normalization, b) definition of the final activity or potency of individual compounds based on a single or a few HCS readouts, and c) generation of hit lists by simple filtering rules.
While such a procedure is suitable for many production screens with a well-defined biology, it is not suitable for either phenotypic screens or MOA studies. In both cases, possible experimental end points are yet to be defined or are simply not obtainable a priori.
Here, we show strategies and results obtained by applying a comprehensive HCS analysis workflow based on image analysis, data pre-processing, and data mining methods. State-of-the-art visualization techniques facilitate the review of data and classification results. Using public benchmark datasets, we show approaches for the de-novo detection of phenotypes and typical downstream analytical questions like classification tasks. We also discuss the trade-offs of object-level vs. well-level analysis in view of computation speed and result quality.
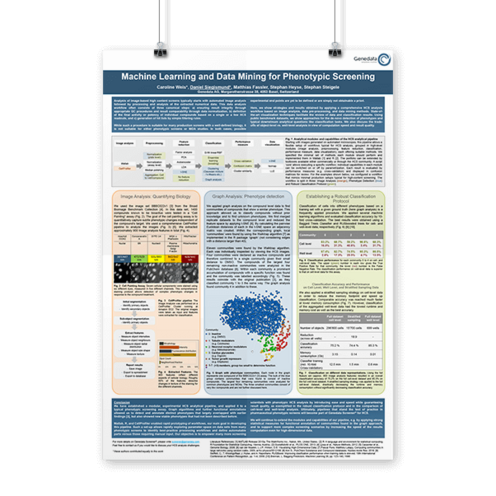