Automated Patchwise Sampling During Deep Learning-Based Image Analysis
Biomolecular Imaging and Informatics 2023, Boston, MA, USA
October 30, 2023
Machine learning based classification of high-content imaging data requires training data, which often requires human curation--including object detection based on segmented cellular components (e.g. the nuclei) identified in the image. Here, we explore a fully automated, patched-based sampling method to systematically create patches from an image, independent of image content or biologically-defined cellular features. This approach offers advantages over segmentation-based methods, including reduced human bias introduced during the curation process and increased flexibility of assay design. However, the optimal parameterization of the patches for training of a deep learning classifier is not clear. Here, we report on the effect of different patch sizes on a deep learning-based image analysis of a myofibroblast differentiation assay. We evaluate performance by considering plate QC statistics, dose-response fitting, and potency (qAC50), comparing these results to a conventional segmentation-based image analysis workflow. These results provide valuable insights into the impact of patch size on model quality and suggest good starting conditions for implementing a rolling-window object definition to train deep learning classifiers.
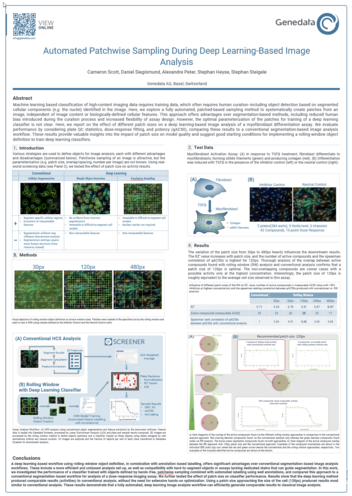